Evaluation of risk factors in patients with breast cancer in stages III and IV: comparison of Cox and Fine-Grey competing risk regression models
DOI:
https://doi.org/10.15419/bmrat.v5i02.417Keywords:
Breast cancer, Competing Risk, Cox regressionAbstract
Introduction: The aim of this study is to fit Fine-Grey competing risk model and compare its results with stratified Cox model and to examine its application in breast cancer data.
Methods: The study was conducted on 15830 women diagnosed with breast cancer in British Columbia, Canada. They were divided into four groups according to patients' stage of disease then for patients with stage III and IV breast cancer was fitted Cox's model and Fine-Grey competing risk flexible models to each group.
Results: The data show that Out of 1888 patients, 578 lied in the age group of below 50 years old, while 1310 were above 50 years of age. The results obtained from fitting stratified Cox regression model indicate that the variables of age and surgery are significant. The patients in the age group of below 50 years old have 70% less hazard in comparison with people older than 50 years of age (HR=0.83). Further, the patients receiving surgery have 38% less hazard in comparison with the patients not receiving surgery (HR=0.62). Then we fit Fine-Grey competing risk models. the variable of chemotherapy is significant in both parametric and semi-parametric competing risk models, and its hazard ratio is HR=1.15 and HR=1.14 in the two models, respectively. On the other hand, the variable of age has not become significant in any of the models, and its hazard ratio is HR=0.92 and HR=0.93, respectively. The variable of surgery in the competing risk parametric model is significant with an HR of 0.67. In Cox model, the variable of surgery is also significant with HR=0.62. Moreover, the variable of age in the competing risk parametric model has not become significant (HR=0.92), and in contrast the variable of age in the Cox model is significant (HR=0.83).
Conclusion: The results of this study show that Considering the comparison of the two models, it is observed that regardless of the properties of competing risk data, estimations of hazard ratio and the extent of significance resulting from Cox models are different from those of competing risk models.
References
Azizi, F., Hatami, H., & Janghorbani, M. (2000). Epidemiology and control of common diseases in Iran (pp. 602–616). Tehran: Eshtiagh Publications.
Baghestani, A. (2014). Gohari M2, Orooji A1, etc. Estimation of Prediction Error for Survival Time with Application in Colorectal Cancer., 22, 157.
Berry, S. D., Ngo, L., Samelson, E. J., & Kiel, D. P. (2010). Competing risk of death: An important consideration in studies of older adults. Journal of the American Geriatrics Society, 58(4), 783–787. https://doi.org/10.1111/j.1532-5415.2010.02767.x PMID:20345862
Chapman, J. A. W., Meng, D., Shepherd, L., Parulekar, W., Ingle, J. N., Muss, H. B., . . . Goss, P. E. (2008). Competing causes of death from a randomized trial of extended adjuvant endocrine therapy for breast cancer. Journal of the National Cancer Institute, 100(4), 252–260. https://doi.org/10.1093/jnci/djn014 PMID:18270335
Cox, D. R., & Oakes, D. (1984). Analysis of survival data. Seconded. London: Chapman and Hall Press.
Gichangi, A., & Vach, W. (2005). The analysis of competing risks data: A guided tour. John Wiley & Sons.
Gutierrez, R.G. (2009). Competing-risks regression -risks regression.
Gutierrez, R.G. (2010). Competing-risks regression -risks regression.
He, X., Esteva, F. J., Ensor, J., Hortobagyi, G. N., Lee, M.-H., & Yeung, S.-C. (2012). Metformin and thiazolidinediones are associated with improved breast cancer-specific survival of diabetic women with HER2+ breast cancer. Annals of Oncology : Official Journal of the European Society for Medical Oncology, 23(7), 1771–1780. https://doi.org/10.1093/annonc/mdr534 PMID:22112968
Klein, J. P. (2006). Modelling competing risks in cancer studies. Statistics in Medicine, 25(6), 1015–1034. https://doi.org/10.1002/sim.2246 PMID:16435339
Latouche, A., & Porcher, R. (2007). Sample size calculations in the presence of competing risks. Statistics in Medicine, 26(30), 5370–5380. https://doi.org/10.1002/sim.3114 PMID:17955563
Lim, H. J., Zhang, X., Dyck, R., & Osgood, N. (2010). Methods of competing risks analysis of end-stage renal disease and mortality among people with diabetes. BMC Medical Research Methodology, 10(1), 97. https://doi.org/10.1186/1471-2288-10-97 PMID:20964855
McPherson k, S.C.M., Dixon J.M. (2000). Abc of breast diseases, breast cancer-epidemiology, risk factors, and genetics. BMJ 321, 625-628.
Nottage, M. K., Kopciuk, K. A., Tzontcheva, A., Andrulis, I. L., Bull, S. B., & Blackstein, M. E. (2006). Analysis of incidence and prognostic factors for ipsilateral breast tumour recurrence and its impact on disease-specific survival of women with node-negative breast cancer: A prospective cohort study. Breast Cancer Research, 8(4), R44. https://doi.org/10.1186/bcr1531 PMID:16859523
Patnaik, J. L., Byers, T., DiGuiseppi, C., Dabelea, D., & Denberg, T. D. (2011). Cardiovascular disease competes with breast cancer as the leading cause of death for older females diagnosed with breast cancer: A retrospective cohort study. Breast Cancer Research, 13(3), R64. https://doi.org/10.1186/bcr2901 PMID:21689398
Pfeiffer, R. M., Park, Y., Kreimer, A. R., Lacey, J. V., Jr., Pee, D., Greenlee, R. T., . . . Hartge, P. (2013). Risk prediction for breast, endometrial, and ovarian cancer in white women aged 50 y or older: Derivation and validation from population-based cohort studies. PLoS Medicine, 10(7), e1001492. https://doi.org/10.1371/journal.pmed.1001492 PMID:23935463
Pintilie, M. (2006). Competing risks: a practical perspective (Vol. 58). John Wiley & Sons. https://doi.org/10.1002/9780470870709
Robson, M. E., Chappuis, P. O., Satagopan, J., Wong, N., Boyd, J., Goffin, J. R., . . . Foulkes, W. D. (2004). A combined analysis of outcome following breast cancer: Differences in survival based on BRCA1/BRCA2 mutation status and administration of adjuvant treatment. Breast Cancer Research, 6(1), R8–R17. https://doi.org/10.1186/bcr658 PMID:14680495
Saadat , S. (2010). Cox proportional hazards regression models to compare and Alan collective survival analysis of breast cancer data (Tehran: shahid Beheshti University of Medical Sciences).
Scheike, T. H., & Zhang, M. J. (2008). Flexible competing risks regression modeling and goodness-of-fit. Lifetime Data Analysis, 14(4), 464–483. https://doi.org/10.1007/s10985-008-9094-0 PMID:18752067
Taghipour, S., Banjevic, D., Fernandes, J., Miller, A. B., Montgomery, N., Harvey, B. J., & Jardine, A. K. S. (2012). Incidence of invasive breast cancer in the presence of competing mortality: The Canadian National Breast Screening Study. Breast Cancer Research and Treatment, 134(2), 839–851. https://doi.org/10.1007/s10549-012-2113-6 PMID:22689090
Tai, B. C., Grundy, R. G., & Machin, D. (2010). On the importance of accounting for competing risks in pediatric cancer trials designed to delay or avoid radiotherapy: I. Basic concepts and first analyses. International Journal of Radiation Oncology, Biology, Physics, 76(5), 1493–1499. https://doi.org/10.1016/j.ijrobp.2009.03.035 PMID:19577860
Tai, B. C., Wee, J., & Machin, D. (2011). Analysis and design of randomised clinical trials involving competing risks endpoints. Trials, 12(1), 127. https://doi.org/10.1186/1745-6215-12-127 PMID:21595883
Varadhan, R., Weiss, C. O., Segal, J. B., Wu, A. W., Scharfstein, D., & Boyd, C. (2010). Evaluating health outcomes in the presence of competing risks: A review of statistical methods and clinical applications. Medical Care, 48(6, Suppl), S96–S105. https://doi.org/10.1097/MLR.0b013e3181d99107 PMID:20473207
Wolbers, M., Koller, M. T., Witteman, J. C., & Steyerberg, E. W. (2009). Prognostic models with competing risks: Methods and application to coronary risk prediction. Epidemiology (Cambridge, Mass.), 20(4), 555–561. https://doi.org/10.1097/EDE.0b013e3181a39056 PMID:19367167
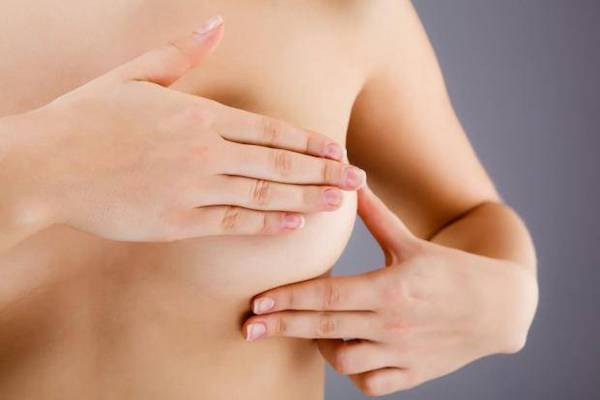
Downloads
Published
Issue
Section
License
Copyright The Author(s) 2017. This article is published with open access by BioMedPress. This article is distributed under the terms of the Creative Commons Attribution License (CC-BY 4.0) which permits any use, distribution, and reproduction in any medium, provided the original author(s) and the source are credited.